Cybersecurity takes a leap forward with AI tools and techniques
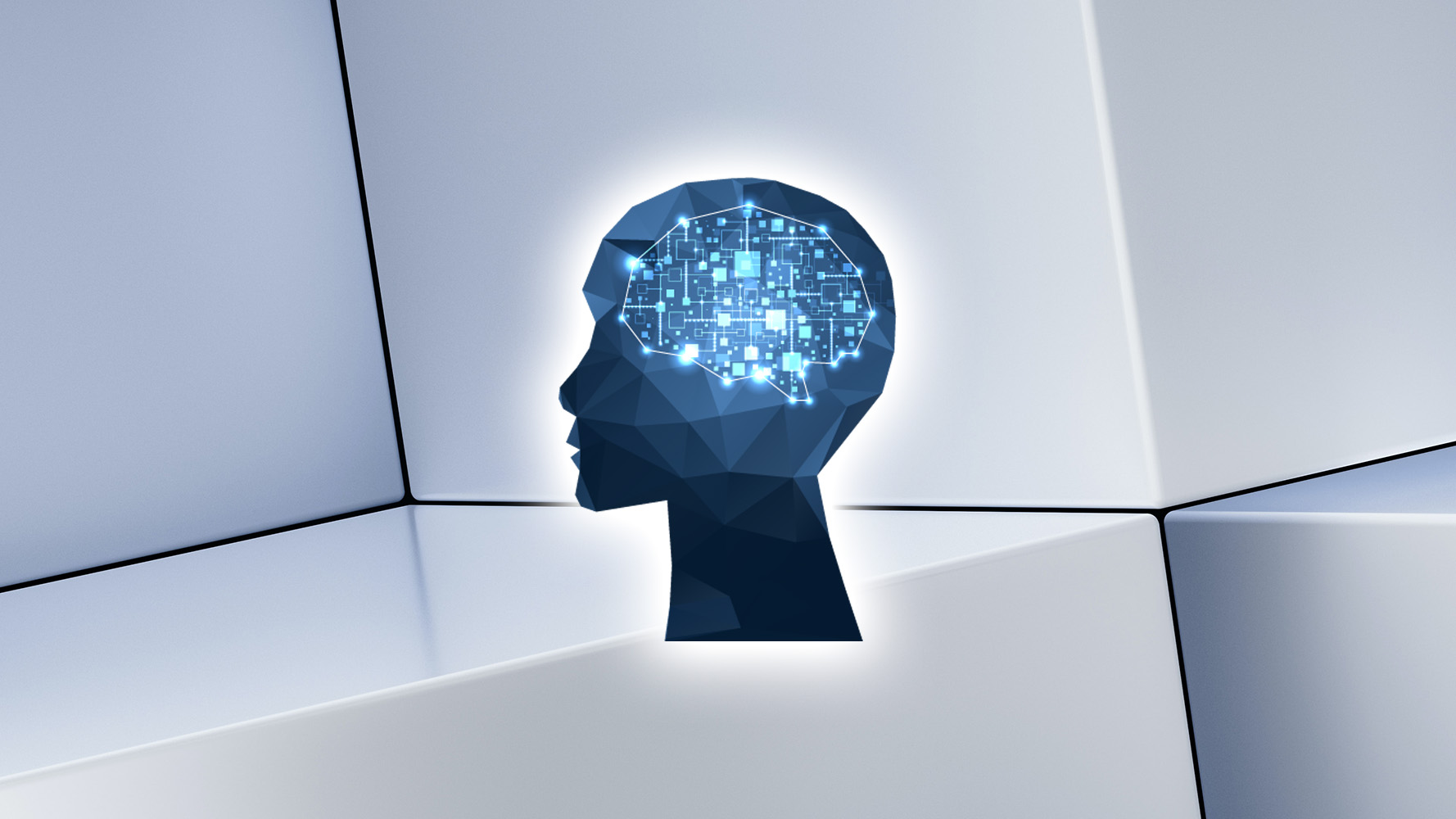
Experts have taken a crucial action towards harnessing a variety of artificial intelligence acknowledged as deep reinforcement mastering, or DRL, to protect personal computer networks.
Autonomus cyber defense framework
When confronted with complex cyberattacks in a demanding simulation location, deep reinforcement understanding was successful at stopping adversaries from reaching their ambitions up to 95 per cent of the time. The outcome features promise for a part for autonomous AI in proactive cyber protection.
Experts from the Office of Energy’s Pacific Northwest National Laboratory (PNNL) documented their findings in a analysis paper.
The starting up position was building a simulation ecosystem to check multistage assault eventualities involving distinct kinds of adversaries. The development of such a dynamic assault-defense simulation environment for experimentation by itself is a win. The setting makes it possible for scientists to assess the performance of unique AI-centered defensive solutions less than controlled take a look at options.
These resources are crucial for evaluating the general performance of deep reinforcement mastering algorithms. The technique is emerging as a powerful conclusion-help instrument for cybersecurity experts – a protection agent with the capability to study, adapt to immediately modifying circumstances, and make selections autonomously. While other sorts of artificial intelligence are normal to detect intrusions or filter spam messages, deep reinforcement discovering expands defenders’ talents to orchestrate sequential choice-earning programs in their daily encounter-off with adversaries.
Deep reinforcement finding out gives smarter cybersecurity, the skill to detect improvements in the cyber landscape before, and the option to choose preemptive steps to scuttle a cyberattack.
DRL: Conclusions in a wide assault space
“An efficient AI agent for cybersecurity requires to feeling, understand, act and adapt, based mostly on the info it can collect and on the results of choices that it enacts,” claimed Samrat Chatterjee, a data scientist who introduced the team’s function. “Deep reinforcement learning holds fantastic opportunity in this area, where by the selection of technique states and action options can be massive.”
DRL, which brings together reinforcement learning and deep understanding, is especially adept in scenarios in which a sequence of conclusions in a elaborate natural environment need to have to be produced. Fantastic selections leading to fascinating effects are strengthened with a optimistic reward (expressed as a numeric benefit) undesirable selections main to undesirable outcomes are discouraged by using a detrimental expense.
It is comparable to how people today find out quite a few duties. A youngster who does their chores could acquire positive reinforcement with a preferred playdate a youngster who doesn’t do their perform gets unfavorable reinforcement, like the takeaway of a electronic system.
“It’s the identical notion in reinforcement understanding,” Chatterjee claimed. “The agent can pick from a established of steps. With just about every motion will come feed-back, excellent or undesirable, that becomes part of its memory. There’s an interplay among discovering new opportunities and exploiting previous encounters. The purpose is to produce an agent that learns to make very good choices.”
MITRE ATT&CK and Open AI Health club
The staff utilised an open up-source program toolkit recognized as Open AI Gym to build a tailor made and controlled simulation environment to appraise the strengths and weaknesses of 4 deep reinforcement understanding algorithms.
They also applied the MITRE ATT&CK framework and included 7 methods and 15 strategies deployed by a few unique adversaries. Defenders had been equipped with 23 mitigation actions to halt or stop an attack’s progression.
The stages of the attack integrated techniques of reconnaissance, execution, persistence, protection evasion, command and command, assortment and exfiltration (when knowledge is transferred out of the system). An assault was recorded as a earn for the adversary if they efficiently reached the last exfiltration phase.
“Our algorithms function in a aggressive environment—a contest with an adversary intent on breaching the technique,” mentioned Chatterjee. “It’s a multistage assault, in which the adversary can pursue multiple assault paths that can improve over time as they try to go from reconnaissance to exploitation. Our challenge is to display how defenses based mostly on deep reinforcement discovering can end these types of an attack.”
DQN (Deep Q-Community)
The workforce educated defensive brokers based mostly on four deep reinforcement studying algorithms: DQN and three variants of what’s regarded as the actor-critic tactic. The brokers have been qualified with simulated info about cyberattacks, then examined from attacks that they experienced not noticed in teaching. DQN performed the ideal.
The very least advanced assaults (based mostly on various ranges of adversary skill and persistence): DQN stopped 79 per cent of assaults halfway through attack stages and 93 % by the last stage.
Reasonably complex assaults: DQN stopped 82 per cent of assaults midway and 95 percent by the final phase.
Most refined attacks: DQN stopped 57 % of assaults halfway and 84 % by the remaining stage—far larger than the other a few algorithms.
“Our target is to generate an autonomous protection agent that can discover the most very likely future step of an adversary, plan for it, and then answer in the greatest way to defend the program,” Chatterjee said.
Regardless of the progress, no just one is ready to entrust cyber defense solely up to an AI method. In its place, a DRL-based cybersecurity method would require to perform in concert with people, mentioned coauthor Arnab Bhattacharya, previously of PNNL.
“AI can be fantastic at defending versus a distinct tactic but isn’t as excellent at being familiar with all the techniques an adversary may well acquire,” Bhattacharya mentioned. “We are nowhere in the vicinity of the phase exactly where AI can change human cyber analysts. Human feedback and steering are vital.”
In addition to Chatterjee and Bhattacharya, authors of the AAAI workshop paper include things like Mahantesh Halappanavar of PNNL and Ashutosh Dutta, a previous PNNL scientist. The get the job done was funded by DOE’s Workplace of Science.